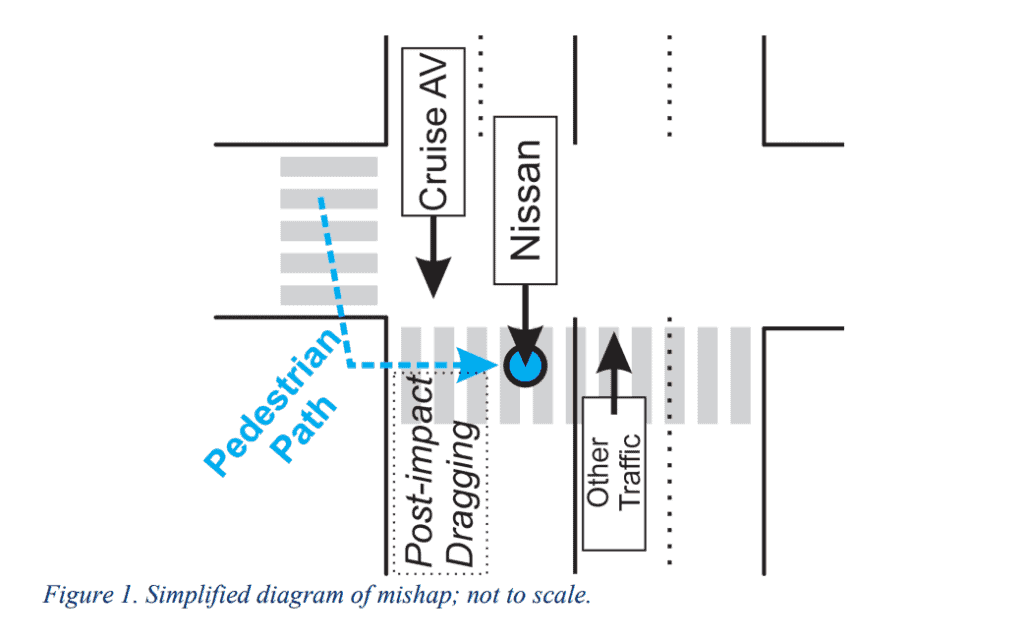
The intersesction in downtown San Francisco where the crash happened ( source: Phil Koopman)
What’s at stake:
Cruise released third-party findings on the Cruise Oct. 2nd crash, offering a semblance of what went wrong. At stake is what Cruise (and other AV companies) will do next. Cruise claims that “based on our internal analysis, we have updated the software to address the underlying issues and filed a voluntary recall with the National Highway Traffic Safety Administration.” Is this enough to make AVs surprise-proof?
We have seen, heard, read, and wondered what exactly happened in the Cruise crash on October 2nd in San Francisco. So far, we know that a woman was first hit by a human-driven car (Nissan) in the lane beside a driverless Cruise. As the Nissan fled, the victim toppled into the path of Cruise robotaxi, which promptly hit her again and dragged her – trapped beneath the vehicle – for 20 feet.
Cruise (and General Motors) funded two recent reports, one by Quinn Emanual Trial Lawyers and another by Exponent, and based on their findings, Phil Koopman, safety expert and professor of Carnegie Mellon University, reconstructed the crash. His report, published last week, is entitled “Anatomy of a Robotaxi Crash: Lessons from the Cruise Pedestrian Dragging Mishap.”
In a podcast interview with The Ojo-Yoshida Report, Koopman stressed that his report is “not my interpretation of what I think happened. This is my reformatting and retelling the story in a more accessible way of what [happened with] Cruise.”
What did the Cruise robotaxi see, when did it see it, and what did it perceive it saw?
We now better understand what went right, and what went wrong.
The questions we need to ask recalls the Watergate query: “What did the president know, and when did he know it?”
In the Cruise case, we ask: What did the Cruise robotaxi see, when did it see it, what did it perceive it saw, what assumptions and predictions did it make, and what actions did it take based on its decisions?
Do AVs ‘understand’?
As Koopman unpacked the timeline, it’s clear that the advanced sensory data collected by the vehicle and the powerful processing capability in the computer driver didn’t help the fully autonomous vehicle “understand” its traffic dilemma or — absent some very specific programming — how to react.
Autonomous vehicles have a pile of scenarios, and they know what to do if they know what to do. And if they don’t know what to do, they just ignore the potential consequences.
Phil Koopman
Nor does the AI-driven vehicle come with common sense.
Koopman explained, “Autonomous vehicles have a pile of scenarios, and they know what to do if they know what to do. And if they don’t know what to do, they just basically ignore the potential consequences.”
Koopman calls this a “design gap.”
In an IEEE Spectrum article published last summer, Missy Cummings, professor at George Mason University, explained that the AI in vehicles is based on the same principles as ChatGPT and other large language models (LLMs). She wrote: “Neither the AI in LLMs nor the one in autonomous cars can ‘understand’ the situation, the context, or any unobserved factors that a person would consider in a similar situation. The difference is that while a language model may give you nonsense, a self-driving car can kill you.”
What did Cruise robocar see, and when did it know?
Koopman’s report, as mentioned, began with two reports commissioned by Cruise. The report written by Quinn Emanuel Trial Lawyers focuses on how Cruise interacted with regulatory bodies. The Exponent (EXPR) text, ”Technical Root Cause Analysis,” was attached as an appendix to the Quin Emanuel Report (QER). Both firms used data collected by the Cruise vehicle. Nonetheless, some portions of both reports, released by Cruise, are heavily redacted.
Koopman explained, “While the QER and EXPR have several timelines and additional information in various places, I integrated the timelines to show some relationships and considerations not readily apparent in the source material.”
The new reports expose critical elements of the accident which were previously neither well understood nor discussed.
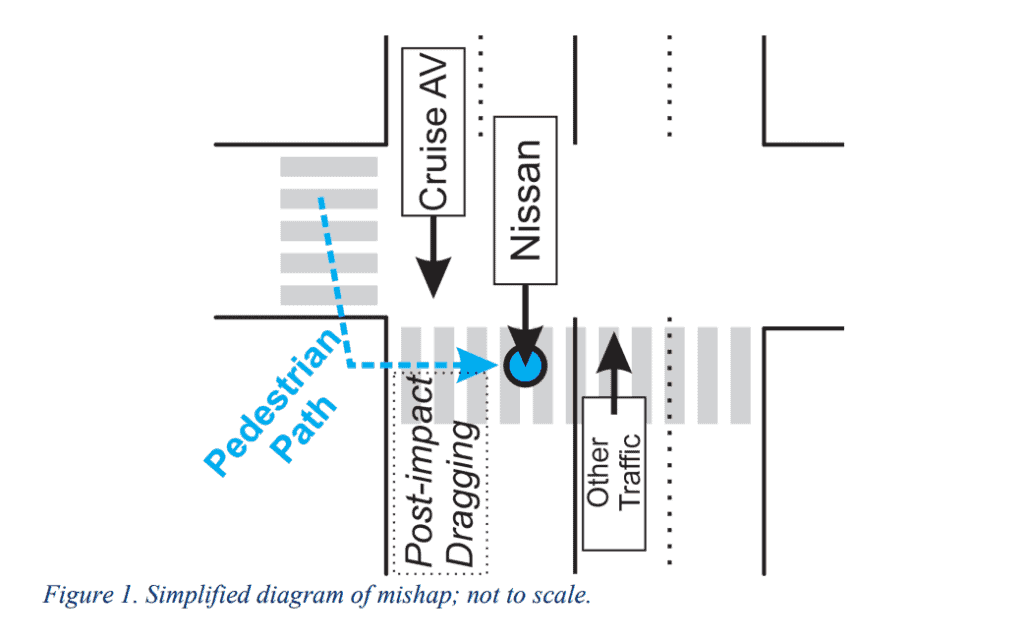
Why accelerate toward a pedestrian?
It turns out that the Cruise AV spotted a pedestrian well before she reached the intersection and entered the marked crosswalk.
Curiously, “The AV accelerated the entire time that it detected a pedestrian in a crosswalk in its travel lane, with speed more than doubling (8 mph increase) during the 2.6 seconds the pedestrian was in its travel lane,” Koopman pointed out.
Why accelerate?
“This was due to the AVs machine learning-based (EXPR 29) prediction that the pedestrian would be out of the way by the time the AV arrived (EXPR 44).”
Koopman noted that “the AV potentially violated California Rules of the Road,” by accelerating toward a pedestrian in a crosswalk. The rule explicitly says that you’re supposed to decelerate to reduce risk of collision.
Tracking the adjacent lane
The Cruise robotaxi was also tracking remarkably well what was going on in the adjacent lane until the human-driven Nissan hit the pedestrian. What was happening only a few feet away, however, was not recognized as an impending collision by the AV, nor did it occur to the AV that this was something to worry about.
Koopman wrote, “It was feasible for the AV to have stopped much sooner, even if only reacting to the pedestrian intrusion into the AV’s lane, avoiding or mitigating initial impact injuries.”
Pedestrian’s feet and lower legs were visible
Third, it was previously unreported that “the pedestrian was not thrown completely onto the ground at impact, but rather initially was at least partly on the hood of the AV before being run over,” Koopman noted.
After the AV ran over the pedestrian, “The pedestrian’s feet and lower legs were visible in the wide-angle side camera view throughout the event (EXPR 83),” Koopman quoted Exponent’s report. In other words, the AV had sensor information showing the pedestrian’s presence under the vehicle, but failed to recognize the scenario, he explained.
Vanishing pedestrian
Then, there’s the phenomenon Koopman calls “the vanishing pedestrian.”
It seems inappropriate to ‘forget’ a pedestrian who has just been hit by a vehicle a few feet away.
Phil Koopman
He wrote: “The AV acted in a way consistent with ‘forgetting’ a pedestrian was in the vicinity. The AV did not stop because of a tracked pedestrian in its lane, but rather because there was occupied road space immediately in front of it that it assumed could be a vulnerable road user. It seems inappropriate to ‘forget’ a pedestrian who has just been hit by a vehicle a few feet away. Moreover, there was sensor information showing the pedestrian, but the system was not up to the tracking challenge.”
Asked what he meant by “occupied space,” Koopman explained, “it knew something was there, but did not have a classification for what was there. However, it was programmed that an undifferentiated ‘something’ should be treated as maybe a pedestrian/VRU (which was a good design decision).”
Koopman said in the podcast that by recognizing the unclassified object as ‘occupied space,’ “Cruise absolutely did the right thing.” By assuming that the occupied space is a vulnerable road user, the Cruise AV knew it had to apply its brakes.
But here’s the rub.
Even though the AV’s sensors had information that would lead to the impeding collision between Nissan and pedestrian, the tracking information at a crucial moment was muddled.
Koopman noted that the last time the AV had the correct classification of a pedestrian was 0.3 seconds before the collision (EXPR 15, 79). “Intermittent classification and tracking of pedestrian resulted in AV detecting ‘occupied space,’ leading to steering and braking commands (EXPR 15, 79).
Despite having recent information that a pedestrian was likely to be struck, “muddled” information, caused the Cruise to lose track of the pedestrian, resulting in the AV’s misdiagnosis of a side-impact collision.
Stopping the car in time?
Once hit by the Nissan, the pedestrian fell into the AV’s lane. The AV started braking too late, hitting the pedestrian at close to maximum pre-impact speed before braking quickly. The AV’s front wheel ran over the pedestrian, leaving the pedestrian under the AV, wrote Koopman. “The laws of physics did not preclude stopping in time to avoid AV/pedestrian impact.”
Quoting Exponent’s report, Koopman summed up the situation:
The AV had enough time to totally avoid impact if it had immediately responded to the pedestrian presence in its lane, even accounting for braking latency (EXPR 66). A delayed but earlier braking “would have potentially mitigated severity of the initial collision” (EXPR 66). Due to a slower than possible response, AV aggressive braking beyond 0.5g did not occur until after impact time 0 (fig. 2).
Minimal Risk Condition
What made the accident truly worse, however, was what happened next.
After the stop, the vehicle initiates a so-called “Minimal Risk Condition.”
This was triggered due to “incorrectly assessing the situation as a side impact rather than a run-over scenario (EXPR 16).”
Throughout this Minimal Risk Maneuver, the pedestrian was trapped under the vehicle, dragged along the pavement for 20 feet at speeds up to 7.7 mph. It could have been worse, Koopman believes. “The AV might have continued this dragging situation for up to 100 feet (QER 14). However, the AV recognized a vehicle motion anomaly and stopped the motion prematurely without recognizing a trapped pedestrian.”
Several issues need attention.
First, a Minimal Risk Condition Maneuver doesn’t necessarily mean that the vehicle must find a curb to stop. “It’s a relative statement,” said Koopman.
As a reporter, I have heard engineers explaining that this Minimal Risk Condition Maneuver is what you’re supposed to do to put the car in a safe spot. Koopman said, “That’s not entirely true. You could stop in lane, and that could also be a minimal risk condition. The definition permits that. The definition needs to be improved.”
But the idea of a minimal risk condition assumes that you’re a traffic hazard and you need to get out of the way. “So if you lose control of your brakes, if you lose control of steering, if you hit something, if your lidar fails, if anything bad happens, you should bring the car to a safe, stopped state. So, a safe, stopped state is minimal risk condition,” Koopman explained.
Generally, Cruise has been criticized severely for stranding cars in the middle of the road and blocking things. “Now, the report doesn’t talk about this, but there’s clearly engineering incentive to get the car out of the travel lane so they don’t get yelled at again. And I get that.”
Koopman speculates that Cruise was trying to aggressively automate getting the car out of the way. “That’s responsive to a lot of stakeholders like the fire trucks who got blocked. But in this case, there was a pedestrian under the vehicle and their computer did not know there was a pedestrian.”
The lesson, Koopman pointed out, is that “automating post-collision actions requires robust sensing and somewhat different prediction capabilities during and after collision.”
Safety engineering
Missing from the official reports – QER and EXPR – was investigation by a real safety engineering expert, Koopman lamented. Cruise initially promised to do so by creating the third report, but later, decided to forgo that idea by expanding the scope of Exponent’s remit “to include a comprehensive review of the company’s safety systems and technology.”
Safety engineering is a ‘parallel activity integrated in the process, in which you ask, we know we are not supposed to hit a pedestrian, but what if we did?’
Any crash reconstruction concludes with changes that could be implemented. But missing from the Cruise analysis, in Koopman’s opinion, is “how do you prevent it from happening again, at a high level safety engineering process level?”
Saying, for example, that “it would be nice to have a sensor here” isn’t what safety engineering analysis does.
Asked for differences between system and safety engineering, Koopman said that in system engineering, engineers list system requirements and they check if the system meets those requirements. But, said Koopman, “by doing good system engineering … you might be missing safety requirements.”
Safety engineering is “this parallel activity integrated in the process, in which you ask, we know we are not supposed to hit a pedestrian, but what if we did? That’s a hazard, and you need to do a hazard analysis.”
Bottom line:
The tech industry, which has spent a decade pitching advancements in sensor capabilities, rapid progress in machine learning and faster reaction time enabled by ever more powerful processors, successfully created its narrative that “self-driving cars are far safer than human-driven cars.” The post-crash analysis of the Cruise accident exposes the AV industry’s denial of a “design gap” that should force it to get serious about “safety engineering.”
Editor’s Note:
The Ojo-Yoshida Report is running a two-part series on this topic with this piece (part 1) focused on technology leading up to the crash. The part 2, written by George Leopold, looks at Cruise’s operations during and after the crash, and responses with regulators. We also offer a full one-hour walkthrough analysis on the Cruise crash by Phil Koopman via our video podcast – Chat with Junko and Bola.
Junko Yoshida is the editor in chief of The Ojo-Yoshida Report. She can be reached at junko@ojoyoshidareport.com.
Copyright permission/reprint service of a full Ojo-Yoshida Report story is available for promotional use on your website, marketing materials and social media promotions. Please send us an email at talktous@ojoyoshidareport.com for details.